Common Misconceptions About Machine Learning and AI
30 Oct 24
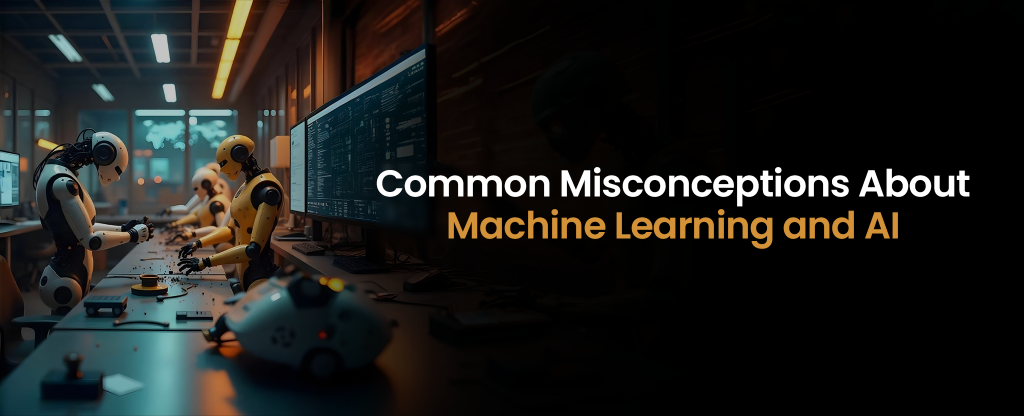
Artificial Intelligence (AI) and Machine Learning (ML) have become buzzwords in recent years, capturing the imagination of people worldwide. However, with their growing popularity comes a fair share of misconceptions. This blog aims to clear up some common misunderstandings about AI and ML, helping you gain a more accurate perspective on these fascinating fields.
AI and Machine Learning Are the Same Thing
One of the most widespread misconceptions is that AI and Machine Learning are interchangeable terms. While they’re closely related, they’re not the same.
- Artificial Intelligence is a broad field of computer science focused on creating intelligent machines that can perform tasks that typically require human intelligence.
- Machine Learning is a subset of AI. It’s a method of teaching computers to learn from data, without being explicitly programmed.
Think of AI as the goal (creating intelligent machines), and ML as one of the ways to achieve that goal.
AI Will Soon Surpass Human Intelligence in All Areas
Movies and science fiction often depict AI as rapidly evolving to surpass human intelligence in every aspect. This idea, known as Artificial General Intelligence (AGI), is still far from reality.
Current AI systems are examples of Narrow AI or Weak AI. They’re designed to perform specific tasks and excel in those areas, but they lack the versatility of human intelligence. For instance:
- An AI might beat the world’s best chess player but wouldn’t know how to play checkers unless specifically trained for it.
- A language model can write coherent text but doesn’t truly understand the meaning behind the words.
While AI continues to advance rapidly, creating a system with human-like general intelligence remains a significant challenge that we’re not close to solving yet.
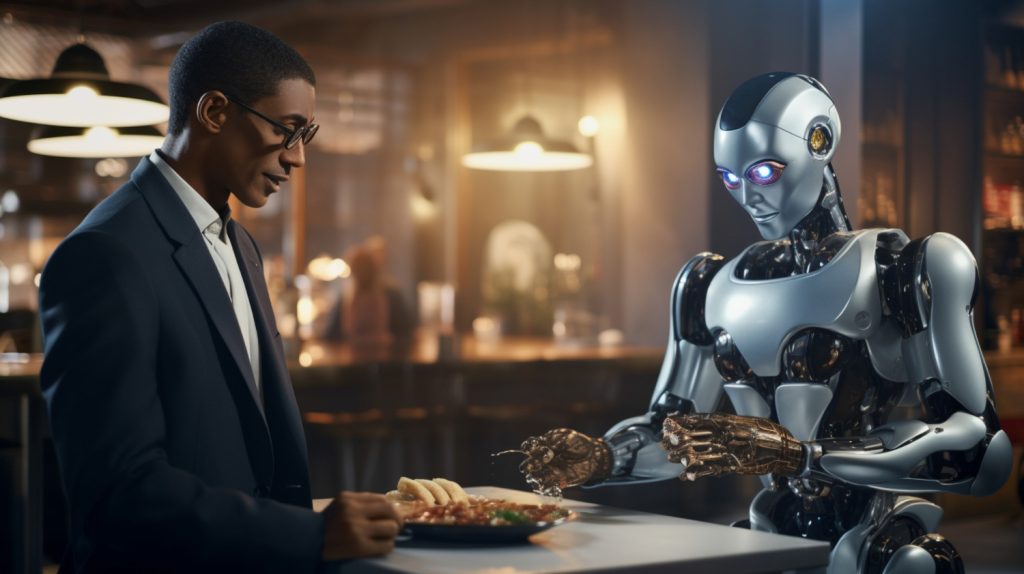
Machine Learning Models Are Always Accurate
There’s a common belief that machine learning models, once trained, always produce accurate results. This is far from the truth. ML models can make mistakes, show biases, or produce unexpected outputs, especially when faced with data different from what they were trained on.
Factors affecting ML model accuracy include:
- Quality and quantity of training data
- Choice of algorithm
- Model parameters
- Complexity of the problem
It’s crucial to remember that ML models are tools that require ongoing monitoring, evaluation, and refinement to maintain their performance.
AI and ML Will Replace All Human Jobs
While it’s true that AI and ML are changing the job market, the idea that they will replace all human jobs is an overstatement. Instead, these technologies are more likely to augment human capabilities and change the nature of work.
Some jobs may become obsolete, but new roles are also emerging:
- AI specialists
- Data scientists
- Machine learning engineers
- AI ethicists
Moreover, many jobs require uniquely human skills like emotional intelligence, creativity, and complex problem-solving, which AI currently struggles to replicate.
Also read Build Your Own AI Model from Scratch: The Ultimate Guide
AI Systems Can Learn and Improve on Their Own
Another common misconception is that once an AI system is deployed, it can continue learning and improving on its own. While some AI systems can learn from new data, this process is usually carefully controlled and supervised by humans.
Most AI systems in use today:
- Are trained on a fixed dataset
- Have their learning “frozen” before deployment
- Require human intervention to update or improve
Continuous learning AI systems do exist, but they’re not as common and come with their own challenges, such as potential degradation of performance over time if not properly managed.
AI Understands Information Like Humans Do
When we see AI systems generating human-like text or recognizing objects in images, it’s easy to assume they understand information the way we do. However, AI systems process information very differently from human brains.
- AI systems work by recognizing patterns in data, not by understanding concepts.
- They don’t have common sense or general knowledge unless it’s explicitly provided in their training data.
- Their “knowledge” is limited to the specific domain they’re trained in.
For example, a language model might produce a coherent paragraph about elephants, but it doesn’t truly understand what an elephant is or have any real-world experience with elephants.
Machine Learning Can Solve Any Problem
While machine learning is a powerful tool, it’s not a magic solution for every problem. There are many challenges that ML is not well-suited to address, especially those requiring:
- Causal reasoning
- Ethical decision-making
- Handling completely novel situations
ML works best when:
- There’s a large amount of high-quality, relevant data available
- The problem can be clearly defined
- There’s a clear relationship between inputs and desired outputs
It’s important to recognize ML’s limitations and use it as part of a broader toolkit for problem-solving.
AI Systems Are Objective and Unbiased
There’s a misconception that because AI systems are machines, they must be objective and free from bias. In reality, AI systems can reflect and even amplify human biases present in their training data or introduced by their creators.
Examples of AI bias:
- Facial recognition systems performing poorly on certain ethnic groups
- Resume screening tools favoring candidates with male-sounding names
- Language models generating text with gender or racial stereotypes
Addressing bias in AI is an ongoing challenge that requires diverse teams, careful data selection, and continuous monitoring and adjustment of AI systems.
You Need to Be a Math Genius to Work in AI or ML
While a strong foundation in mathematics is certainly helpful in AI and ML, you don’t need to be a math prodigy to work in these fields. There are many roles in AI and ML that require different skill sets:
- Data collection and preparation
- Model deployment and maintenance
- AI ethics and policy
- User experience design for AI-powered products
Moreover, with the development of user-friendly ML tools and platforms, it’s becoming increasingly accessible for people with diverse backgrounds to work with AI and ML technologies.
AI Will Solve All of Humanity’s Problems
Some people view AI as a panacea that will solve all of humanity’s problems. While AI has the potential to help address many challenges, it’s not a silver bullet. AI is a tool, and like any tool, its impact depends on how we use it.
AI can contribute to solving problems in areas like:
- Healthcare (disease diagnosis, drug discovery)
- Climate change (energy optimization, climate modeling)
- Education (personalized learning)
However, many of our most pressing issues also require political will, social change, and human decision-making that can’t be outsourced to AI.
Conclusion
As AI and ML continue to evolve and impact our lives, it’s crucial to separate fact from fiction. By understanding what these technologies can and cannot do, we can better utilise their potential while being aware of their limitations.
Remember:
- AI and ML are powerful tools, but they’re not infallible or all-encompassing.
- They augment human capabilities rather than completely replacing them.
- Ethical considerations and human oversight remain crucial in the development and deployment of AI systems.
By dispelling these common misconceptions, we can approach AI and ML with a more balanced and informed perspective, enabling us to make better decisions about how to develop and use these technologies in the future.
- Android Development2
- Artificial Intelligence15
- Classified App1
- Digital Transformation7
- Doctor Appointment Booking App10
- Ecommerce Apps22
- Education Apps2
- Fintech-Apps30
- Flutter2
- Flutter Apps19
- Food Delivery App5
- Grocery Apps2
- Health Care4
- IoT2
- Loyalty Programs8
- Microsoft1
- Mobile App Maintenance1
- Mobile Apps117
- Product Engineering2
- Progressive Web Apps1
- Saas Application2
- Shopify2
- Software Development1
- Taxi Booking Apps7
- Truck Booking App5
- UI UX Design8
Comments